정리 기준은 다음과 같다.
1. is. zero-shot? few-shot? full-shot?
2. method? SAM? GPT? CLIP? MIXED?
3. trainable? non-trainable?
+ 뭘 바꿔야 AD성능이 올라갈까?
- 논문을 쭉 읽으면서 느낀 부분은 시작은 항상 CLIP으로 한다. 클립으로 되는걸 SAM으로 하면 성능이 더 좋아진다. 그리고 SAM이나 CLIP으로 한걸 GPT가 이해하는 그런 방향으로 논문이 반복되는 것 같다.
어떻게 정리할까
1. 서베이를 자세히 읽는다. 2025년도 서베이 기준으로, 포인트는 FM으로 잡는다.
2. 서베이 기준으로 FM 논문을 찾는다.
3. 논문을 요약할 땐,
- 이전 연구 문제점
- 개선사항
- method
- setting을 본다.
Survey
1. Beyond Academic Benchmarks: Critical Analysis and Best Practices for Visual
Industrial Anomaly Detection
2. A Survey on Foundation-Model-Based Industrial Defect Detection
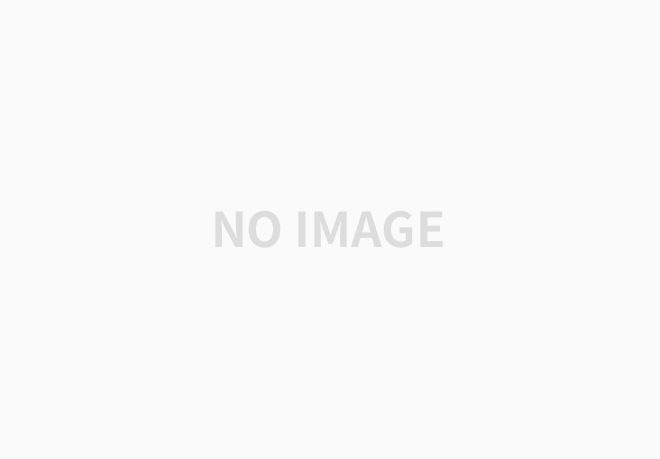
3. Foundation Models for Anomaly Detection: Vision and Challenges
논문 찾다 보니 MVTec 새로 나온거 발견함
The MVTec AD 2 Dataset: Advanced Scenarios
for Unsupervised Anomaly Detection
[읽은 논문]
Text-Guided Variational Image Generation for Industrial Anomaly Detection and Segmentation
Towards Training-free Anomaly Detection with Vision and Language Foundation Models
Toward Generalist Anomaly Detection via In-context Residual Learning with Few-shot Sample Prompts
MetaUAS: Universal Anomaly Segmentation with One-Prompt Meta-Learning
WinCLIP: Zero-/Few-Shot Anomaly Classification and Segmentation
AnomalyGPT: Detecting Industrial Anomalies Using Large Vision-Language Models
FastRecon: Few-shot Industrial Anomaly Detection via Fast Feature Reconstruction
PromptAD: Learning Prompts with only Normal Samples for Few-Shot Anomaly Detection
AnomalyCLIP: Object-agnostic Prompt Learning for Zero-shot Anomaly Detection
VCP-CLIP: A Visual Context Prompting Model for Zero-shot Anomaly Segmentation
AA-CLIP: Enhancing Zero-shot Anomaly Detection via Anomaly-Aware CLIP
AdaCLIP: Adapting CLIP with Hybrid Learnable Prompts for Zero-Shot Anomaly Detection
Towards Zero-Shot Anomaly Detection and Reasoning with Multimodal Large Language Models
One-for-All Few-Shot Anomaly Detection via Instance-Induced Prompt Learning ------- [x]
MuSc: Zero-Shot Industrial Anomaly Classification and Segmentation with Mutual Scoring of the Unlabeled Images
UniVAD: A Training-free Unified Model for Few-shot Visual Anomaly Detection
[남은 논문]
Exploring Intrinsic Normal Prototypes within a Single Image for Universal Anomaly Detection
Bayesian Prompt Flow Learning for Zero-Shot Anomaly Detection
Anomaly Heterogeneity Learning for Open-set Supervised Anomaly Detection
Supervised Anomaly Detection for Complex Industrial Images
CableInspect-AD: An Expert-Annotated Anomaly Detection Dataset
Few Shot Part Segmentation Reveals Compositional Logic for Industrial Anomaly Detection
CNC: Cross-modal Normality Constraint for Unsupervised Multi-class Anomaly Detection
Authors
Omni-AD: Learning to Reconstruct Global and Local Features for Multi-class Anomaly Detection